Making sense of the world through data The focus of this blog is #data #bigdata #dataanalytics #privacy #digitalmarketing #AI #artificialintelligence #ML #GIS #datavisualization and many other aspects, fields and applications of data
Monday, March 25, 2019
Rethinking Internet Advertising
"I got the feeling we're not in Kansas anymore!" And just like that, by opening a door, Dorothy brought "color" to cinema and the world in 1939.
Unfortunately, this watershed moment has not taken place on the Internet yet: We are still on television! Or so advertisers seem to believe.
The metrics have changed, we count clicks and measure engagement now but the rest is the same, pathetically similar in fact as advertisers try to squeeze their true and proven TV formats on our smaller, interactive Internet screens with utmost disregard to the viewers' experience and the effect on brands.
But who can blame them? 50 years of advertising cornucopia on TV have made them soft. How can you change what has worked so well for so long? Surly, the 30 second spot must be the ultimate ad experience to insure that consumers reach for the recognized names when they select products in supermarkets' alleys?
Or could it be that our world is truly changing and that not only brick and mortar stores are on the way out but the full advertising ecosystem which fed brand recognition to shoppers is going too? A true paradigm shift unrecognized in its amplitude and scope as they usually are?
But then again, how is it possible that what is supposed to be the most creative part of our society, the out-of-the-box thinkers are all taken wrong footed, pushing the wrong messages the wrong way on a media they do not understand well, alienating viewers instead of wooing them?
To answer this question, we need to understand better the Internet, the way it works, it's impact on people and ultimately to rethink advertising.
Originally advertising is nothing more than commercial information and as such it needed a name (before it became a brand), an address and a product. This gave us the beautiful streets of traditional European cities with their easily recognizable shop signs as in Salzburg.
With the industrial revolution and print media, things became more complicated. People could not see anymore what you were doing so you needed to lure them. Usually a few words and an address would do. But slowly the inserts became more sophisticated with better design and longer messages crowding the front and back pages of newspapers and magazines. True advertising had arrived.
Then, soon after the first world war, radio came of age and with it the second dimension of advertising: Time. The duration of your message was now of critical importance. You needed to cram as much information in as little time as possible. Slogans worked best.
And finally television in the 1950s. It took a surprisingly long time to build up the post-war consumer society and for all the cogs in the machine to move smoothly. Early TV ads are often little more than a still newspaper picture with a radio text read in the background. Then TV commercials were refined and the TV spot was born for mass audience with precise targeting and effective messaging.
But once the system was in place, it went on and on. Like the time machine traveler, The world changed around advertising but advertising did not change much over the years. Finally, the Internet spread in the 1990s and with it out of nowhere, the new ad giants, Google and Facebook were born. Because they were pioneers, they could do no wrong. Their strength vindicated by the new metrics they created to actually measure the impact of advertising: CPM (Cost per thousand), CPC (Cost per Click) and CPA (Cost per Action).
But the real challenge was to manage the infinite but highly diluted real estate of the Internet and more specifically how to grab the attention of viewers which at 9 seconds flat was said to be less than that of a goldfish!
And this is how we ended up with what cannot be called anything but pollution and garbage on our screens: Banners, promoted outstream videos, sponsored contents, forever "jumping" in-feed ads (to make sure you click on them by "accident") and the rest. A jungle of sneaky (native advertising) or obstructive ideas designed to pull attention and distract by any means available. Each new idea being worse than the one before with an arm race for our dwindling attention at war with our natural organic defenses embodied by ad blockers.
In fact, over the last few years, the average Internet experience has become truly awful with a ceaseless barrage of ads and interruption to the point that older less savvy people are pushed out of the Internet outright.
Clearly something has gone wrong. But what can we do about it?
The first step is to recognize that the Internet is not television and that what worked well with a passive audience on TV cannot be replicated or achieve the same results with an interactive pull media such as the Internet.
You now need to engage people with your brand or message to actually reach your audience. The challenge of course is how to do this.
We haven't found a universal solution yet because there are none! Given the opportunity, different people behave differently and understanding the dynamic nature of the Internet and the fact that people are not the passive audience they used to be helps.
This in fact changes everything!
Shouting your brands name in bold characters on your target's screen, interrupting their reading, following them with pesky ads from page to page with incessant calls to action are the best way to discover the interactive nature of the Internet with your message being muted or worse shut off completely. A feedback which has cleverly been obfuscated by Internet platforms.
But this does not mean that advertising is dead and condemned to be counter-productive from now on. Quite the opposite in fact. But to fully take advantage of the Internet, advertisers must understand better how their targets behave and are clustered by the opportunities offered.
The reason why this has not happened yet is due to the overwhelming presence of the Internet giants who in their rush for profits and over-reliance on one media only, have been unable or rather unwilling to understand the profound revolution that the Internet represents and the fact that with it, advertising has entered a new dimension of communication where instead of replacing the former media as was the case until now, the Internet is transcending them all and can be used to unify communication on disparate platforms.
Consequently, what brands need, more than an "Internet strategy", is to rethink how to engage with their clients in a more proactive way across platforms and touch points. A far more daunting challenge which explains why so few have been up to the task yet.
So if you cannot splash your "name" on the right "segments" screens, how can you reach your audience in the Internet age?
Simply, you are back to the basics of marketing, trying to understand your audience, its tastes and likes, what turns them on and off. Their needs and expectations. their aspirations and how to answer them.
In this respect, the Nike campaign for the 2018 winter Olympic games in Korea is interesting to my opinion as it shows a possible way forward. They sponsored evens and stars the usual way to display the brand during the games but then expanded their scope after the games by actually creating new events such as marathons where thousands of people participated, paid for their gear, generating a buzz online.
This is the difference between a pop-up on your screen seen as an interruption which will irritate you and a message from a friend on WhatsApp asking you if you want to participate to a race and sending you a couple of pictures after the event: Soft pressure!
The move to a true multi-platform advertising experience is on-going. It will be a complex solution adapted to multiple behaviors and segments. Advertisers will exchange your information for actual insight. Soften or harden the intensity of their messaging, add AI to analyze feedback and adapt faster. It is quite likely that we ain't seen nothing yet as advertising finally adjust to the Internet.
Saturday, March 23, 2019
Social Media trends in 2019
Over the coming weeks, we will be exploring the trends in Social media and Internet use in 2019, looking at the wider world while focusing on Japan and APAC.
Most of the information comes from the analysis of Simon Kemp at Kepios https://kepios.com/
who every year published his Digital report in Asia highlighting the main factors shaping and defining our future.
Once you are past the fact that Simon has kept a smattering of accent from the country of craigs and lochs where they swear at the rain all the way to the local pub, there is no denying that the insight of data he offers is profound and helps understand what comes next.
So what comes next?
Here's two insights we will be looking at in depth:
1 - Internet advertising stinks!
It took 10 years for advertisers to understand television and the fact that you needed to address viewers in a new way. More specifically that the still pictures of newspapers and the audios of radio were not the right way to engage their new audience on TV.
Unfortunately it looks like the conceptual gap between TV and Internet is wider and consequently the adaptation will be harder.
The pathetic supplications to turn off your add blockers will cut no ice with people overwhelmed with an oversupply of outstanding material available on demand.
Splashing your brand's name in bothering inserts on the screen of unwilling viewers is not a recipe for success as the reaction is most likely to be negative while turning off future engagement with the brand.
Advertising on the Internet must be reinvented.
We will explore how some companies like Nike in Korea do this successfully by creating events where thousands of people not only pay to participate but then eagerly share their experience on social media.
2 - Fake News everywhere!
One of 2018 buzz words was "Fake News" as if news were ever meant to be "true" or even "news"! But now we know and the world will never be the same again.
At the same time as people get locked even deeper in echo chambers of their own making by filtering out whatever information seems dissonant with their feelings, emotions and knowledge, they have become more wary and suspicious of outside sources accelerating the trend towards general skepticism and conversely the ready acceptation of dubious information relentlessly pegged by networks with an agenda.
One case in point: Facebook and their dramatic loss of audience.
After each of the multiple scandals which rocked Facebook last year we have seen countless articles trending on the Internet outlining the precipitous "fall" of engagement on Facebook. Unfortunately, the data does not support this at all! Facebook actual reach in 2018 kept growing by almost 9%. The only demographic which went down was very young people who seems to be switching to other platforms. Concerning but not cataclysmic. And it is not even a new trend as this has been going on for some time now. What was reported on social medias and in the news was simply not true or to put it more bluntly: wishful thinking supported by no data whatsoever, i.e. "lies"!
We will dive on this subject too with specific data to understand what is going on and figure out the trends from the noise. Facebook is definitively not out of the woods but they are not sinking either... yet!
https://kepios.com/blog/2018/11/27/the-future-of-social-why-the-basics-are-still-essential?fbclid=IwAR0u5CYVil44z3Nenbz6BO80Q7p-2jPCluk9_-ltN1m5azYp95P7SUcv75s
Wednesday, March 20, 2019
The march of the algorithm (Weekend cartoon)
Math is perfect but language is not!
This is why the Universe must speak the language of mathematics.
There is no other way.
Here is the difference:
In this particular example, the question should be:
"What is the value of X in this triangle?"
Likewise, a complex society must speak the language of algorithm.
There is no other way.
It is the same joke as in mathematics where here the student just replicates the pattern as a "copy" instead of creating it as a program.
This answers the question in a narrow sense which is of course not what the question was.
This is why, slowly algorithm are taking over. Sector by sector, function by function, they are slowly inserting their invisible presence and ruthless efficiency in our everyday world until one day our smart cars will move in a smart city with no humans left in sight.
There will be no room left for jokes, everything will be "efficient".
Perfect answers to perfect questions!
But is this the world we want to live in?
A world run by AI runs smoothly but it has no room for us.
Sunday, March 17, 2019
What can AI actually do for you in marketing?
What can Artificial Intelligence actually do for you in marketing?
Not tomorrow, today!
Not so much yet and certainly not as much as the hype we read on a daily basis.
AI is just not ready yet for prime time. But it doesn't mean that some limited solutions are useless, quite the contrary as explained below in the article from MIT Sloane Management Review.
The five applications are:
1 - Lead scoring and predictive analytics
This is an obvious application of AI because although marketeers can do it with whatever data feedback they have at hand, AI can take more data into consideration and improve its accuracy quickly by measuring complex correlations.
2 - Automated e-mail conversation
This is a rather risky application because of the blow back risk from clients as soon as they realize they are not being engaged by a real human.
3 - Customer Insights
This requires an in-depth understanding of what AI is and is not. In a nutshell, AI is not yet "intelligence" per se but only a limited aspect of it called Machine Learning. This means that AI cannot yet dive much deeper into "data" than a human counterpart but can actually analyses what a human cannot such as voice tone and emotions. But this is most certainly not a "simple" application easily accessible to any company.
4 - Personalizing with data
Yes the amount of data has exploded in recent years but this is more often a curse than a blessing: What is relevant and what is not? Can a AI software "find" unknown correlations in your data? Maybe but it is not obvious. Then can the software find interesting information in "context" data such as a whole industry? Again, maybe but you still have to feed the AI with the right data.
5 - Content creation
We are still very far from it, but as support for templates, subjects and a list of "do and don't" AI certainly can play a role to enhance productivity.
Here is the article with a more detailed explanation for each example:
https://sloanreview.mit.edu/article/five-ai-solutions-transforming-b2b-marketing/
#statistics #DataScience #data #DataAnalytics #AI #ArtificialIntelligence #ML
Mapping voices and emotions
I have always been fascinated how mapping technology can be used outside its realm of geography to display information and show a different aspect of data inaccessible to raw number analysis in order to extract information at a higher level.
This study is a perfect example that shows how our voice can be use as proxy data to display our emotions.
The challenge is to use the right syntax to make the data understandable and meaningful. The information can then be corroborated with known facts to confirm or infirm hypothesis and advance knowledge.
Here, the science study from the University of Berkeley states that:
"Previous studies had pegged the number of emotions we can express with vocal bursts at around 13. But when the UC Berkeley team analyzed their results, they found there are at least 24 distinct ways that humans convey meaning without words.
“Our findings show that the voice is a much more powerful tool for expressing emotion than previously assumed,” said study lead author Alan Cowen, a psychology graduate at UC Berkeley, in a press release.
http://blogs.discovermagazine.com/d-brief/2019/02/12/vocal-bursts-human-sounds-communicate-wordless/?utm_source=dscfb&utm_medium=social&utm_campaign=dscfb&fbclid=IwAR3ytWS10nBwMW4hPo9PltauBVB-qSp9t0GXthWllY3RaTLH8p_1U0ouET4#.XI7PSLiRU2w
Thursday, March 14, 2019
How to chose the number of clusters in a model?
After a quick reminder of how it should be done theoretically from an article in Data Science Central (The full article can be found below) https://www.datasciencecentral.com/profiles/blogs/how-to-automatically-determine-the-number-of-clusters-in-your-dat
We will explain how we actually do it in the real world of marketing below.
1 - The theory of determining clusters
Determining the number of clusters when performing unsupervised
clustering is a tricky problem. Many data sets don't exhibit well
separated clusters, and two human beings asked to visually tell the
number of clusters by looking at a chart, are likely to provide two
different answers. Sometimes clusters overlap with each other, and large
clusters contain sub-clusters, making a decision not easy.
For instance, how many clusters do you see in the picture below? What is the optimum number of clusters? No one can tell with certainty, not AI, not a human being, not an algorithm.
In the above picture, the underlying
data suggests that there are three main clusters. But an answer such as 6
or 7, seems equally valid.
A number of empirical approaches have
been used to determine the number of clusters in a data set. They
usually fit into two categories:
For instance, how many clusters do you see in the picture below? What is the optimum number of clusters? No one can tell with certainty, not AI, not a human being, not an algorithm.
- Model fitting techniques: an example is using a mixture model to fit with your data, and determine the optimum number of components; or use density estimation techniques, and test for the number of modes (see here.) Sometimes, the fit is compared with that of a model where observations are uniformly distributed on the entire support domain, thus with no cluster; you may have to estimate the support domain in question, and assume that it is not made of disjoint sub-domains; in many cases, the convex hull of your data set, as an estimate of the support domain, is good enough.
- Visual techniques: for instance, the silhouette or elbow rule (very popular.)
Good references on the topic are available. Some R functions are available too, for instance fviz_nbclust. However, I could not find in the literature, how the elbow point is explicitly computed. Most references mention that it is mostly hand-picked by visual inspection, or based on some predetermined but arbitrary threshold. In the next section, we solve this problem.
2 - How we actually find clusters
The article is longer and explains how to actually do it.
https://www.datasciencecentral.com/profiles/blogs/how-to-automatically-determine-the-number-of-clusters-in-your-dat
But in real life, and if you have any understanding of your data at all, this is useless!
It is essential to understand why with a quick example.
In the chart above, the optimum number of clusters is 4. But what if we chose 6 as indicated or 14 as the underlying data in grey seems to indicate? In that case, what we find is that the balance of the clusters (not just the mode) changes quite dramatically, and the 4 original clusters become unrecognizable which is normal since we have a rather large overlap of data.
And unfortunately, the result as indicated by the market (on-line client use) is bad. Our clusters are under-performing.
To solve the problem, we inverted the question: What are the "known" clusters in our population? Whatever the data you work with, there will be some accumulated knowledge about that population. In our case, marketing, clusters are clear and defined by the clients: "Young working women" or "Retired urban couples" for example.
Our task is now simpler and far more accurate: Which "known" clusters can we identify in our data?
This has proved extremely helpful and made our clusters very popular with clients because they recognize them right-away and because they perform well!
The only risk is that often you cannot identify the "known" clusters in you data because of the difficulty to link parameters. In that case the solution is quite simple: Vary your parameters and see how your data distribution changes.
Saturday, March 9, 2019
No, Machine Learning is not just glorified Statistics
No, Machine Learning is not just glorified Statistics
Published on: Towards Data Science
by: Joe Davidson
on: June 27, 2018
https://towardsdatascience.com/no-machine-learning-is-not-just-glorified-statistics-26d3952234e3
This
meme has been all over social media lately, producing appreciative
chuckles across the internet as the hype around deep learning begins to
subside. The sentiment that machine learning is really nothing to get
excited about, or that it’s just a redressing of age-old statistical
techniques, is growing increasingly ubiquitous; the trouble is it isn’t
true.
I
get it — it’s not fashionable to be part of the overly enthusiastic,
hype-drunk crowd of deep learning evangelists. ML experts who in 2013
preached deep learning from the rooftops now use the term only with a
hint of chagrin, preferring instead to downplay the power of modern
neural networks lest they be associated with the scores of people that
still seem to think that
import keras
is the leap for every hurdle, and that they, in knowing it, have some tremendous advantage over their competition.
While it’s true that deep learning has outlived its usefulness as a buzzword, as Yann LeCun put it,
this overcorrection of attitudes has yielded an unhealthy skepticism
about the progress, future, and usefulness of artificial intelligence.
This is most clearly seen by the influx of discussion about a looming AI winter, in which AI research is prophesied to stall for many years as it has in decades past.
The
purpose of this post isn’t to argue against an AI winter, however. It
is also not to argue that one academic group deserves the credit for
deep learning over another; rather, it is to make the case that credit is
due; that the developments seen go beyond big computers and nicer
datasets; that machine learning, with the recent success in deep neural
networks and related work, represents the world’s foremost frontier of
technological progress.
Machine Learning != Statistics
“When you’re fundraising, it’s AI. When you’re hiring, it’s ML. When you’re implementing, it’s logistic regression.”
—everyone on Twitter ever
The
main point to address, and the one that provides the title for this
post, is that machine learning is not just glorified statistics—the
same-old stuff, just with bigger computers and a fancier name. This
notion comes from statistical concepts and terms which are prevalent in
machine learning such as regression, weights, biases, models, etc.
Additionally, many models approximate what can generally be considered
statistical functions: the softmax output of a classification model
consists of logits, making the process of training an image classifier a
logistic regression.
Though
this line of thinking is technically correct, reducing machine learning
as a whole to nothing more than a subsidiary of statistics is quite a
stretch. In fact, the comparison doesn’t make much sense. Statistics is
the field of mathematics which deals with the understanding and
interpretation of data. Machine learning is nothing more than a class of
computational algorithms (hence its emergence from computer science).
In many cases, these algorithms are completely useless in aiding with
the understanding of data and assist only in certain types of
uninterpretable predictive modeling. In some cases, such as in
reinforcement learning, the algorithm may not use a pre-existing dataset
at all. Plus, in the case of image processing, referring to images as instances of a dataset with pixels as features was a bit of a stretch to begin with.
The
point, of course, is not that computer scientists should get all the
credit or that statisticians should not; like any field of research, the
contributions that led to today’s success came from a variety of
academic disciplines, statistics and mathematics being first among them.
However, in order to correctly evaluate the powerful impact and
potential of machine learning methods, it is important to first
dismantle the misguided notion that modern developments in artificial
intelligence are nothing more than age-old statistical techniques with
bigger computers and better datasets.
Machine Learning Does Not Require An Advanced Knowledge of Statistics
Hear me out.
When I was learning the ropes of machine learning, I was lucky enough
to take a fantastic class dedicated to deep learning techniques that was
offered as part of my undergraduate computer science program. One of
our assigned projects was to implement and train a Wasserstein GAN in
TensorFlow.
At
this point, I had taken only an introductory statistics class that was a
required general elective, and then promptly forgotten most of it.
Needless to say, my statistical skills were not very strong. Yet, I was
able to read and understand a paper on a state-of-the-art generative
machine learning model, implement it from scratch, and generate quite
convincing fake images of non-existent individuals by training it on the
MS Celebs dataset.]
Throughout
the class, my fellow students and I successfully trained models for
cancerous tissue image segmentation, neural machine translation,
character-based text generation, and image style transfer, all of which
employed cutting-edge machine learning techniques invented only in the
past few years.
Yet,
if you had asked me, or most of the students in that class, how to
calculate the variance of a population, or to define marginal
probability, you likely would have gotten blank stares.
That seems a bit inconsistent with the claim that AI is just a rebranding of age-old statistical techniques.
True,
an ML expert probably has a stronger stats foundation than a CS
undergrad in a deep learning class. Information theory, in general,
requires a strong understanding of data and probability, and I would
certainly advise anyone interested in becoming a Data Scientist or
Machine Learning Engineer to develop a deep intuition of statistical
concepts. But the point remains: If
machine learning is a subsidiary of statistics, how could someone with
virtually no background in stats develop a deep understanding of
cutting-edge ML concepts?
It
should also be acknowledged that many machine learning algorithms
require a stronger background in statistics and probability than do most
neural network techniques, but even these approaches are often referred
to as statistical machine learning or statistical learning,
as if to distinguish themselves from the regular, less statistical
kind. Furthermore, most of the hype-fueling innovation in machine
learning in recent years has been in the domain of neural networks, so
the point is irrelevant.
Of
course, machine learning doesn’t live in a world by itself. Again, in
the real world, anyone hoping to do cool machine learning stuff is
probably working on data problems of a variety of types, and therefore
needs to have a strong understanding of statistics as well. None of this
is to say that ML never uses or builds on statistical concepts either,
but that doesn’t mean they’re the same thing.
Machine Learning = Representation + Evaluation + Optimization
To
be fair to myself and my classmates, we all had a strong foundation in
algorithms, computational complexity, optimization approaches, calculus,
linear algebra, and even some probability. All of these, I would argue,
are more relevant to the problems we were tackling than knowledge of
advanced statistics.
Machine
learning is a class of computational algorithms which iteratively
“learn” an approximation to some function. Pedro Domingos, a professor
of computer science at the University of Washington, laid out three components that make up a machine learning algorithm: representation, evaluation, and optimization.
Representation involves
the transformation of inputs from one space to another more useful
space which can be more easily interpreted. Think of this in the context
of a Convolutional Neural Network. Raw pixels are not useful for
distinguishing a dog from a cat, so we transform them to a more useful
representation (e.g., logits from a softmax output) which can be
interpreted and evaluated.
Evaluation
is essentially the loss function. How effectively did your algorithm
transform your data to a more useful space? How closely did your softmax
output resemble your one-hot encoded labels (classification)? Did you
correctly predict the next word in the unrolled text sequence (text
RNN)? How far did your latent distribution diverge from a unit Gaussian
(VAE)? These questions tell you how well your representation function is
working; more importantly, they define what it will learn to do.
Optimization is the last piece of the puzzle. Once you have the evaluation component, you can optimize the representation function in order to improve your evaluation metric.
In neural networks, this usually means using some variant of stochastic
gradient descent to update the weights and biases of your network
according to some defined loss function. And voila! You have the world’s
best image classifier (at least, if you’re Geoffrey Hinton in 2012, you
do).
When
training an image classifier, it’s quite irrelevant that the learned
representation function has logistic outputs, except for in defining an
appropriate loss function. Borrowing statistical terms like logistic regression
do give us useful vocabulary to discuss our model space, but they do
not redefine them from problems of optimization to problems of data
understanding.
Aside: The term artificial intelligence is stupid. An AI problem is just a problem that computers aren’t good at solving yet. In the 19th century, a mechanical calculator was considered intelligent (link).
Now that the term has been associated so strongly with deep learning,
we’ve started saying artificial general intelligence (AGI) to refer to
anything more intelligent than an advanced pattern matching mechanism.
Yet, we still don’t even have a consistent definition or understanding
of general intelligence. The only thing the term AI does is inspire fear
of a so-called “singularity” or a terminator-like killer robot. I wish
we could stop using such an empty, sensationalized term to refer to real
technological techniques.
Techniques For Deep Learning
Further
defying the purported statistical nature of deep learning is, well,
almost all of the internal workings of deep neural networks. Fully
connected nodes consist of weights and biases, sure, but what about
convolutional layers? Rectifier activations? Batch normalization?
Residual layers? Dropout? Memory and attention mechanisms?
These
innovations have been central to the development of high-performing
deep nets, and yet they don’t remotely line up with traditional
statistical techniques (probably because they are not statistical
techniques at all). If you don’t believe me, try telling a statistician
that your model was overfitting, and ask them if they think it’s a good
idea to randomly drop half of your model’s 100 million parameters.
And let’s not even talk about model interpretability.
Regression Over 100 Million Variables — No Problem?
Let
me also point out the difference between deep nets and traditional
statistical models by their scale. Deep neural networks are huge. The
VGG-16 ConvNet architecture, for example, has approximately 138 million parameters. How do you think your average academic advisor would respond to a student wanting to perform a multiple regression of over 100 million variables? The idea is ludicrous. That’s because training VGG-16 is not multiple regression — it’s machine learning.
New Frontiers
You’ve
probably spent the last several years around endless papers, posts, and
articles preaching the cool things that machine learning can now do, so
I won’t spend too much time on it. I will remind you, however, that not
only is deep learning more than previous techniques, it has enabled to
us address an entirely new class of problems.
Prior
to 2012, problems involving unstructured and semi-structured data were
challenging, at best. Trainable CNNs and LSTMs alone were a huge leap
forward on that front. This has yielded considerable progress in fields
such as computer vision, natural language processing, speech
transcription, and has enabled huge improvement in technologies like
face recognition, autonomous vehicles, and conversational AI.
It’s
true that most machine learning algorithms ultimately involve fitting a
model to data — from that vantage point, it is a statistical procedure.
It’s also true that the space shuttle was ultimately just a flying
machine with wings, and yet we don’t see memes mocking the excitement
around NASA’s 20th century space exploration as an overhyped rebranding
of the airplane.
As
with space exploration, the advent of deep learning did not solve all
of the world’s problems. There are still significant gaps to overcome in
many fields, especially within “artificial intelligence”. That said, it
has made a significant contribution to our ability to attack problems
with complex unstructured data. Machine learning continues to represent
the world’s frontier of technological progress and innovation. It’s much
more than a crack in the wall with a shiny new frame.
Edit:
Many
have interpreted this article as a diss on the field of statistics, or
as a betrayal of my own superficial understanding of machine learning.
In retrospect, I regret directing so much attention on the differences
in the ML vs. statistics perspectives rather on my central point: machine learning is not all hype.
Let
me be clear: statistics and machine learning are not unrelated by any
stretch. Machine learning absolutely utilizes and builds on concepts in
statistics, and statisticians rightly make use of machine learning
techniques in their work. The distinction between the two fields is
unimportant, and something I should not have focused so heavily on.
Recently, I have been focusing on the idea of Bayesian neural networks. BNNs involve
approximating a probability distribution over a neural network’s
parameters given some prior belief. These techniques give a principled
approach to uncertainty quantification and yield better-regularized
predictions.
I
would have to be an idiot in working on these problems to say I’m not
“doing statistics”, and I won’t. The fields are not mutually exclusive,
but that does not make them the same, and it certainly does not make
either without substance or value. A mathematician could point to a
theoretical physicist working on Quantum field theory and rightly say
that she is doing math, but she might take issue if the mathematician
asserted that her field of physics was in fact nothing more than
over-hyped math.
So
it is with the computational sciences: you may point your finger and
say “they’re doing statistics”, and “they” would probably agree.
Statistics is invaluable in machine learning research and many
statisticians are at the forefront of that work. But ML has developed
100-million parameter neural networks with residual connections and
batch normalization, modern activations, dropout and numerous other
techniques which have led to advances in several domains, particularly
in sequential decision making and computational perception. It has found
and made use of incredibly efficient optimization algorithms, taking
advantage of automatic differentiation and running in parallel on
blindingly fast and cheap GPU technology. All of this is accessible to
anyone with even basic programming abilities thanks to high-level,
elegantly simple tensor manipulation software. “Oh, AI is just logistic
regression” is a bit of an under-sell, don’t ya think?
https://towardsdatascience.com/no-machine-learning-is-not-just-glorified-statistics-26d3952234e3
Friday, March 8, 2019
The Achilles' Heel Of AI
With AI, data preparation which was already important is becoming crucial. The potential to get it wrong is multiplying as the options of what we call data taxonomy increases and many of the steps are not yet standardized as highlighted in the article.
Published in Forbes COGNITIVE WORLD
by Ron Schmelzer
On May 7, 2019
https://www.forbes.com/sites/cognitiveworld/2019/03/07/the-achilles-heel-of-ai/#4cbff5177be7
Garbage in is garbage out. There’s no
saying more true in computer science, and especially is the case with
artificial intelligence. Machine learning algorithms are very dependent on
accurate, clean, and well-labeled training data to learn from so that they can
produce accurate results. If you train your machine learning models with
garbage, it’s no surprise you’ll get garbage results. It’s for this reason that
the vast majority of the time spent during AI projects are during the data
collection, cleaning, preparation, and labeling phases.
According to a recent report from AI
research and advisory firm Cognilytica, over 80% of the time spent in AI
projects are spent dealing with and wrangling data. Even more importantly, and
perhaps surprisingly, is how human-intensive much of this data preparation work
is. In order for supervised forms of machine learning to work, especially the
multi-layered deep learning neural network approaches, they must be fed large
volumes of examples of correct data that is appropriately annotated, or
“labeled”, with the desired output result. For example, if you’re trying to get
your machine learning algorithm to correctly identify cats inside of images,
you need to feed that algorithm thousands of images of cats, appropriately
labeled as cats, with the images not having any extraneous or incorrect data
that will throw the algorithm off as you build the model. (Disclosure: I’m a
principal analyst with Cognilytica)
Data Preparation: More than Just Data
Cleaning
According to Cognilytica’s report, there
are many steps required to get data into the right “shape” so that it works for
machine learning projects:
Removing or correcting bad data and duplicates - Data in the enterprise
environment is exceedingly “dirty” with incorrect data, duplicates, and other
information that will easily taint machine learning models if not removed or
replaced.
Standardizing and formatting data - Just how many different ways are there
to represent names, addresses, and other information? Images are many different
sizes, shapes, formats, and color depths. In order to use any of this for
machine learning projects, the data needs to be represented in the exact same
manner or you’ll get unpredictable results.
Updating out of date information - The data might be in the right format
and accurate, but out of date. You can’t train machine learning systems when
you’re mixing current with obsolete (and irrelevant) data.
Enhancing and augmenting data - Sometimes you need extra data to make
the machine learning model work, such as calculated fields or additional
sourced data to get more from existing data sets. If you don’t have enough
image data, you can actually “multiply” it by simply flipping or rotating
images while keeping their data formats consistent.
Reduce noise - Images, text, and data can have “noise”, which is
extraneous information or pixels that don’t really help with the machine
learning project. Data preparation activities will clear those up.
Anonymize and de-bias data - Remove all unnecessary personally
identifiable information from machine learning data sets and remove all
unnecessary data that can bias algorithms.
Normalization - For many machine learning algorithms, especially
Bayesian Classifiers and other approaches, data needs to be represented in
standard ranges so that one input doesn’t overpower others. Normalization works
to make training more effective and efficient.
Data sampling - If you have very large data sets, you need to sample
that data to be used for the training, test, and validation phases, and also
extract subsamples to make sure that the data is representative of what the real-world
scenario will be like.
Feature enhancement - Machine learning algorithms work by training on
“features” in the data. Data preparation tools can accentuate and enhance the
data so that it is more easily able to separate the stuff that the algorithms
should be trained on from less relevant data.
You can imagine that performing all these
steps on gigabytes, or even terabytes, of data can take significant amounts of
time and energy. Especially if you have to do it over and over until you get
things right. It’s no surprise that these steps take up the vast majority of
machine learning project time. Fortunately, the report also details solutions
from third-party vendors, including Melissa Data, Paxata, and Trifacta that
have products that can perform the above data preparation operations on large
volumes of data at scale.
Data Labeling: The Necessary Human in the
Loop
In order for machine learning systems to
learn, they need to be trained with data that represents the thing the system
needs to know. Obviously, as detailed above that data needs to not only be good
quality, but it needs to be “labeled” with the right information. Simply having
a bunch of pictures of cats doesn’t train the system unless you tell the system
that those pictures are cats -- or a
specific breed of cat, or just an animal, or whatever it is you want the system
to know. Computers can’t put those labels on the images themselves, because it
would be a chicken-and-egg problem. How can you label an image if you haven’t
fed the system labeled images to train it on?
The answer is that you need people to do
that. Yes, the secret heart of all AI systems is human intelligence that labels
the images systems later use to train on. Human powered data labeling is the necessary
component for any machine learning model that needs to be trained on data that
hasn’t already been labeled. There are a growing set of vendors that are
providing on-demand labor to help with this labeling, so companies don’t have
to build up their own staff or expertise to do so. Companies like CloudFactory,
Figure Eight, and iMerit have emerged to provide this capacity to organizations
that are wise enough not to build up their own labor force for necessary data
labeling.
Eventually, there will be a large amount of
already trained neural networks that can be used by organizations for their own
model purposes, or extended via transfer learning to new applications. But
until that time, organizations need to deal with the human-dominated labor
involved in data labeling, something Cognilytica has identified takes up to 25%
of total machine learning project time and cost.
AI helping Data Preparation
Even with all this activity in data
preparation and labeling, Cognilytica sees that AI will have an impact on this
process. Increasingly, data preparation firms are using AI to automatically
identify data patterns, autonomously clean data, apply normalization and
augmentation based on previously learned patterns, and aggregate data where
necessary based on previous machine learning projects. Likewise, machine
learning is being applied to data labeling to speed up the process by
suggesting potential labels, applying bounding boxes, and otherwise speeding up
the labeling process. In this way, AI is being applied to help make future AI
systems even better.
The final conclusion of this report is that
the data side of any machine learning project is usually the most labor
intensive part. The market is emerging to help make those labor tasks less onerous
and costly, but they can never be eliminated. Successful AI projects will learn
how to leverage third-party software and services to minimize the overall cost
and impact and lead to quicker real-world deployment.
Subscribe to:
Posts (Atom)
UK/EU VETO on Trump-Putin talks (Video - 27mn)
It may be just my understanding, but listen carefully between the lines and what you realize is that "if" there is a meeting be...
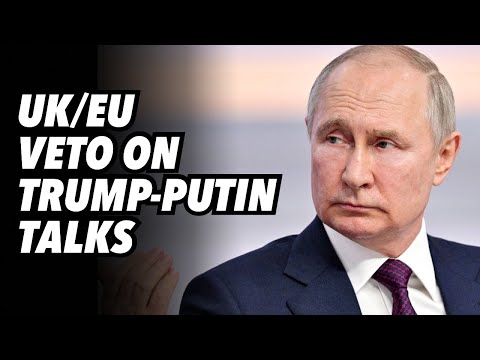
-
A little less complete than the previous article but just as good and a little shorter. We are indeed entering a Covid dystopia. Guest Pos...
-
In a sad twist, from controlled news to assisted search and tunnel vision, it looks like intelligence is slipping away from humans alm...
-
A rather interesting video with a long annoying advertising in the middle! I more or less agree with all his points. We are being ...